In the pursuit of business growth, dedicating valuable time and resources to every lead that comes your way is an inefficient approach. It’s important to differentiate between consumers who have a genuine interest in your products and services and those who don’t. To optimize your marketing and sales efforts, it’s crucial to implement a tailored lead-scoring model. By assessing the value and interest level of each lead, you can prioritize your focus on high-potential prospects, ensuring effective resource allocation and driving meaningful business growth.
What Is Lead Scoring and How Can It Benefit Your Business?
Lead scoring involves assigning values, typically in the form of numerical points, to evaluate the quality and potential of each generated lead for a business. Various attributes such as the lead’s professional information and engagement with the brand online can be taken into account for scoring. By implementing scoring, sales and marketing teams can effectively prioritize leads, tailor their response strategies, and ultimately enhance the conversion rate of leads into customers. This systematic approach optimizes resource allocation and improves overall sales and marketing outcomes.
Lead scoring varies across companies, but leveraging past lead data is a common approach. By analyzing successful customer profiles and non-converting leads, you can establish a value system based on key attributes.
While lead scoring may seem straightforward, complexities can arise based on your business model and database. To simplify the process, we will guide you through the fundamentals of building a lead score. Discover essential data to consider, identify crucial attributes, and learn how to calculate a basic score effectively. Gain insights to streamline your lead scoring efforts and optimize lead management.
Types of Lead Scoring Models
The larger your business is, the more lead-scoring models you need to cover your buyers’ different behaviors and characteristics. Here are three types of models to consider for your lead-scoring marketing strategies.
Types of Lead Scoring Models | Description |
---|---|
Fit vs. Interest | Scoring leads based on their fit to the buyer persona and their interest in the product. Many models combine both scores for the highest value targets. |
Lead Scoring for Different Personas | Different scoring models for each customer persona created, as B2B and B2C customers may show vastly different behaviors. |
Returning Customers | Potential for upgrading or cross-selling, but may not rank well on traditional lead scoring models. An amended model is required for repeat customer behaviors. |
How to Build a Model That Works
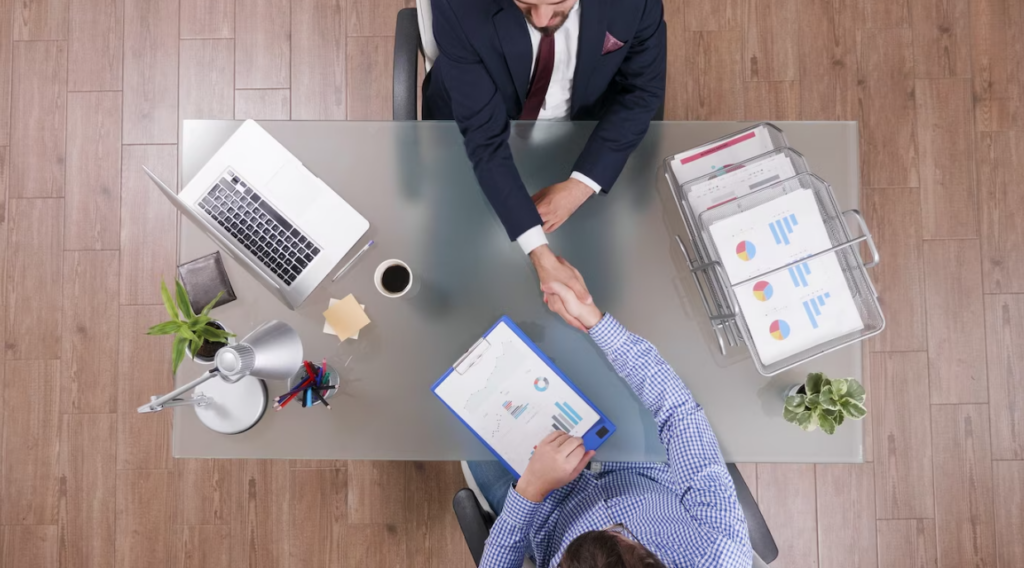
Step 1: Defining Your Ideal Leads
Before implementing a scoring model, it’s crucial to identify the characteristics of your ideal leads. These are the leads that are most likely to benefit from your solution and have a higher chance of converting into customers. Start by examining your existing successful customers and look for commonalities among them. Consider the following questions to refine your understanding:
- What is the company size?
- What is the company’s revenue?
- How many employees are there in the company?
- What job titles do the employees hold?
- Where is the company located?
- What industry or vertical does the company belong to?
- Which specific department or team would benefit from your solution?
- How many employees are typically in that department?
By answering these questions and exploring other relevant factors, you can narrow down and clearly identify your ideal leads. Additionally, collaborating with your marketing team can provide valuable insights from their market research to further define your target audience.
Step 2: Establish Criteria for Qualifying an Ideal Lead
To develop an effective lead scoring model, you need to consider two types of data: demographic information and behavioral interactions with your company.
Demographic Data:
Based on the characteristics you identified in Step 1, you can gather demographic information that helps determine an ideal lead. This data is typically provided by leads when they fill out forms on your website or through conversations you’ve had with them. The following five demographic criteria can be easily identified:
- Job title;
- Industry type;
- Company size;
- Location;
- Department.
Behavioral Data:
The second type of data focuses on the lead’s interactions with your company. It encompasses their actions on your website and engagement with your emails. Positive buying signals to observe include opening and responding to emails, visiting pricing pages, and signing up for your solution. On the other hand, visiting the careers page or unsubscribing from your mailing list may indicate disinterest.
Consider the following positive buying signals in your lead-scoring model:
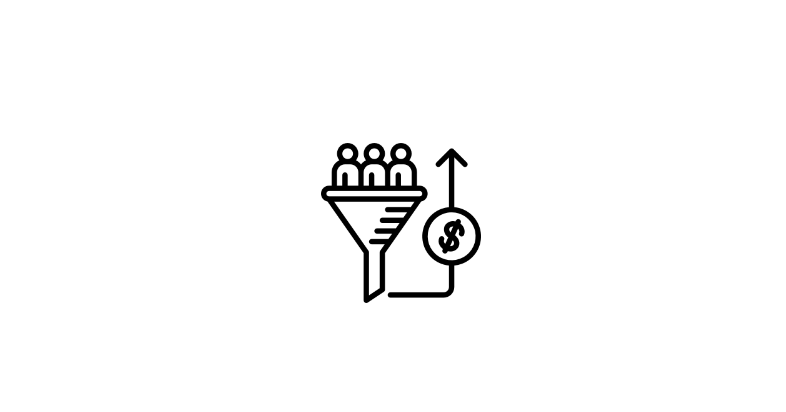
- Top-of-the-funnel activities like requesting a demo or downloading a whitepaper;
- Middle-of-the-funnel activities like visiting pricing pages, registering for product webinars or signing up for your solution;
- Actions performed within your application, such as watching onboarding videos, adding a team, or adopting new features;
- Opening and engaging with company emails or clicking links in the email body.
Both demographic and behavioral data play vital roles in setting up a lead-scoring model. Demographic data indicates your interest in the lead, while behavioral data reflects the lead’s interest in your company. Scoring leads using only one category may fail to differentiate between a disinterested CEO and a manager with a high intent to purchase.
Step 3: Assigning Values
Determining the appropriate scores for each activity is often the most challenging aspect of lead scoring, and it’s where many people make mistakes.
An effective approach is to allocate points equally between demographic and behavioral data. This ensures that leads don’t receive a high score solely based on matching your ideal lead profile or showing surface-level engagement with your brand.
For instance, you may assign 20 points to the CEO of a small business, who aligns with your ideal lead. Conversely, a student visiting your careers page might receive negative points as their behavior indicates a mismatch with your target audience.
Step 4: Establish a Score Threshold
After assigning scores to your leads, it’s important to set a threshold that categorizes leads based on their readiness for sales engagement. Not all leads will fall into the “red-hot” category, as some may not be ready to make a purchase while others may require further nurturing. By defining threshold limits, you can easily identify leads that need immediate attention and those that need ongoing nurturing.
For instance, you can establish the following threshold limits:
Score Threshold Categories | Description |
---|---|
Hot or Sales-Ready Leads | Leads with a score above 70 that are ready for sales engagement. |
Warm Leads | Leads with a score between 70 and 30 that require further nurturing before sales engagement. |
Cold Leads | Leads with a score below 30 that require significant nurturing before sales engagement. |
Step 5: Continuously Evaluate and Enhance Your Lead Scoring Model
As time progresses, it’s essential to regularly evaluate the performance of your lead scoring model. Initially, your scoring rules are based on hypotheses, but after six months or more, you may start observing new trends among your leads. For instance, you might discover increased engagement levels from leads within unexplored industry types or find that leads reaching the sales-ready score are not yet ready to make a purchase. These observations indicate the need to revisit and refine your lead scoring model.
It is advisable to monitor the results of your lead-scoring efforts on an ongoing basis. Stay open to making adjustments, conducting tests, and seeking improvements. Developing an effective lead-scoring model is an iterative process that requires continuous maintenance and refinement.
Manual Lead Scoring
Manual Lead Scoring is a method used by businesses to assess the quality and potential of their leads based on specific criteria and attributes. Unlike predictive scoring, which relies on machine learning and algorithms, manual lead scoring involves a more hands-on approach.
Manual Lead Scoring Steps | Description |
---|---|
Evaluate Lead-to-Customer Conversion Rate | Calculate the lead-to-customer conversion rate by dividing the number of new customers by the total number of leads generated. This rate serves as a benchmark to measure the effectiveness of lead-scoring efforts. |
Identify Key Attributes of Higher Quality Leads | Select specific attributes that are commonly associated with higher quality leads, such as customers who have requested a free trial or customers within a particular industry. Use insights from sales team conversations, analytics, and other relevant data sources to inform attribute selection. |
Determine Individual Close Rates for Each Attribute | Analyze the actions or characteristics that lead to qualified leads and eventual conversions to calculate the close rates for each attribute. This step helps understand the effectiveness of different attributes in driving successful outcomes and is used to assign scores in the next step. |
Compare Attribute Close Rates and Assign Point Values | Compare the close rates of each attribute with the overall close rate and identify attributes that significantly outperform the overall close rate. Assign point values to these attributes based on the magnitude of their individual close rates, ensuring consistency in assigning point values across attributes. For example, if the “requested demo” attribute has a close rate 20 times higher than the overall close rate, assign 20 points to leads with that attribute. |
Logistic Regression Lead Scoring
While the manual approach mentioned above is a great starting point, implementing a more mathematically sound method like logistic regression can enhance accuracy. Logistic regression utilizes data mining techniques to build a formula that predicts the probability of a lead converting into a customer.
By considering the interactions between various customer attributes (such as industry, company size, and trial requests), logistic regression provides a holistic approach to lead scoring. This technique aligns closely with your actual close rates, making it a more precise method.
If you’re interested in exploring logistic regression in Excel, you can refer to the provided resource. However, the manual approach outlined earlier is an effective way to begin your lead-scoring journey.
Predictive Lead Scoring
Implementing a lead scoring system can bring numerous benefits to your business, such as streamlining lead handoff, increasing conversion rates, and boosting sales team productivity. However, manually creating and maintaining a scoring system can be a time-consuming process.
Fortunately, there’s a solution: predictive scoring. By leveraging machine learning, predictive scoring takes the burden off your team by automatically analyzing vast amounts of data points. It identifies patterns among your customers and non-converting leads to develop a formula that ranks your contacts based on their potential to become customers. This technology-driven approach eliminates the need for manual setup and ongoing adjustments, freeing up your team’s time to focus on building customer relationships.
With predictive scoring, you gain valuable insights into common attributes shared by your best leads. This enables you and your sales team to prioritize leads effectively, avoiding unnecessary follow-ups with disinterested prospects while engaging with those who show genuine interest. By harnessing the power of predictive scoring, you can optimize your lead management process and maximize the efficiency of your sales efforts.